Biography
Timeline & Experiences
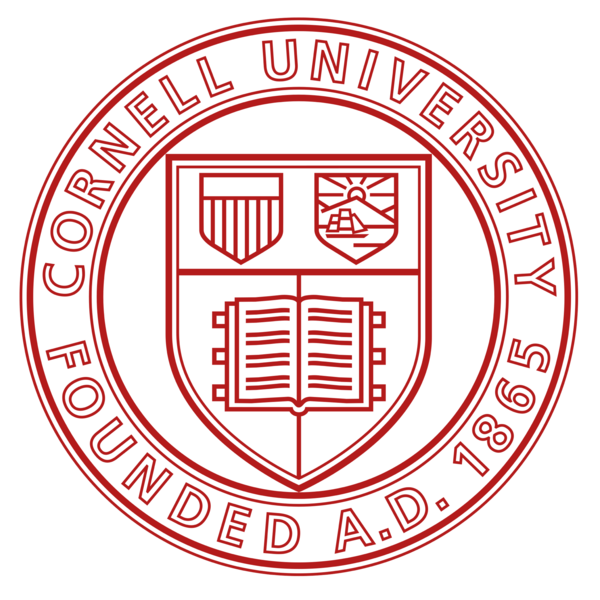
CS Phd student @ Cornell Tech, Cornell University
Machine Learning, Computer Vision, and Graphics
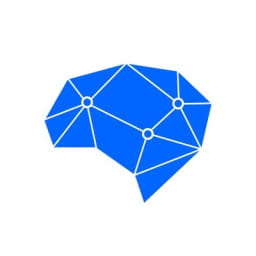
Research Engineer @ Horizon Robotics
Representation Learning, Object-oriented Learning, & Reinforcement Learning
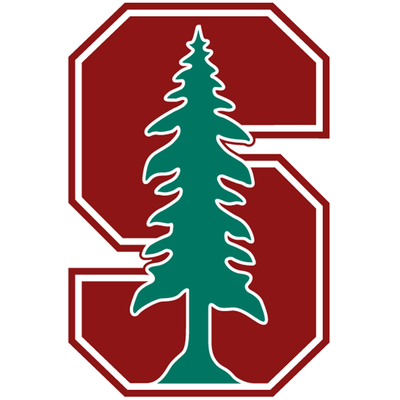
Research Assistant @ Stanford Vision and Learning Lab (SVL)
Computer Vision, Neural Rendering, and Multimodal Learning
Supervisor: Prof. Li Fei-Fei & Prof. Jiajun Wu
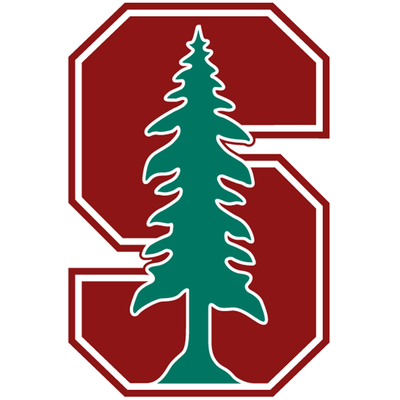
EE master student @ Stanford University
Research Assistant @ Stanford Network Analysis Project (SNAP)
Deep Learning, Graph Mining, Anomaly Detection
Deep Learning, Graph Mining, Anomaly Detection
Supervisor: Prof. Jure Leskovec & Prof. Pan Li
Research Assistant @ Stanford Vision & Learning Lab (SVL)
Computer Vision, Neural Rendering, Multimodal Learning
Computer Vision, Neural Rendering, Multimodal Learning
Supervisor: Prof. Li Fei-Fei & Prof. Jiajun Wu
Graduate Researcher @ Stanford Geometric Computation Group
Computer Vision, 3D learning, CAD Model Analysis
Computer Vision, 3D learning, CAD Model Analysis
Supervisor: Prof. Leonidas Guibas
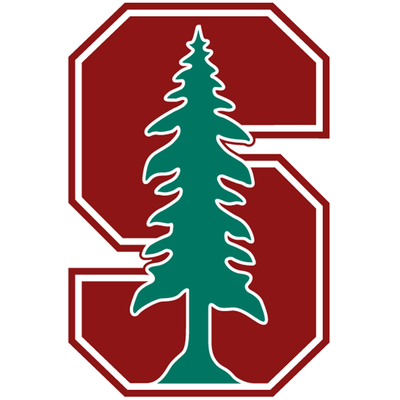
Summer Research Intern @ Stanford Network Analysis Project (SNAP)
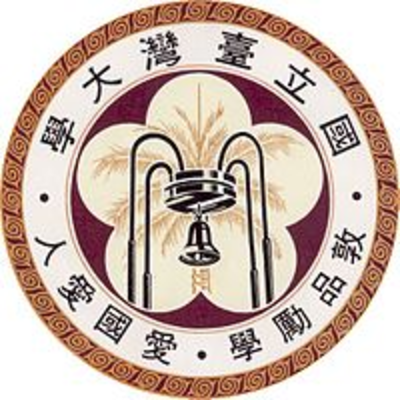
Undergraduate student & researcher @ NTU
Electrical Engineering department
1996
He was born.
Selected Publications
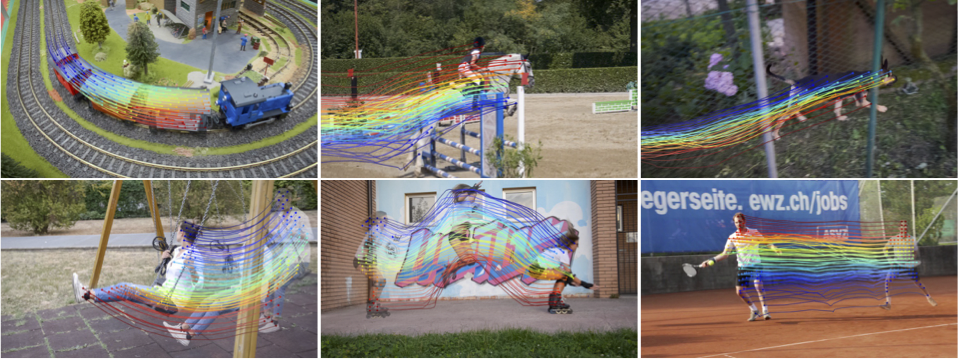
Tracking Everything Everywhere All at Once
ICCV 2023
(Oral)
Paris, France
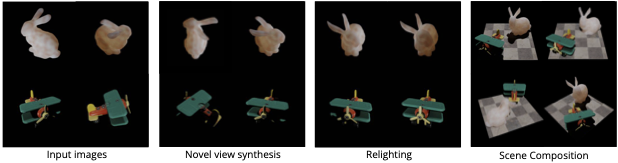
Learning Object-centric Neural Scattering Functions for Free-viewpoint Relighting and Scene Composition
TMLR 2023
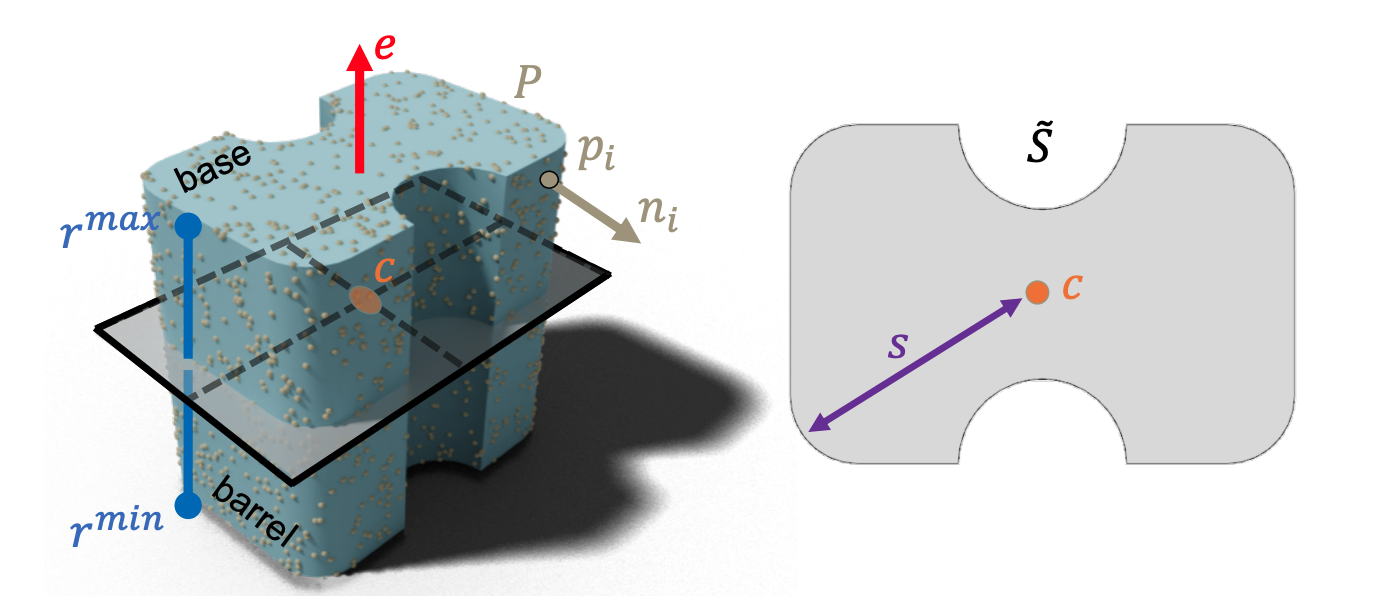
Point2Cyl: Reverse Engineering 3D Objects from Point Clouds to Extrusion Cylinders
CVPR 2022
New Orleans, LA
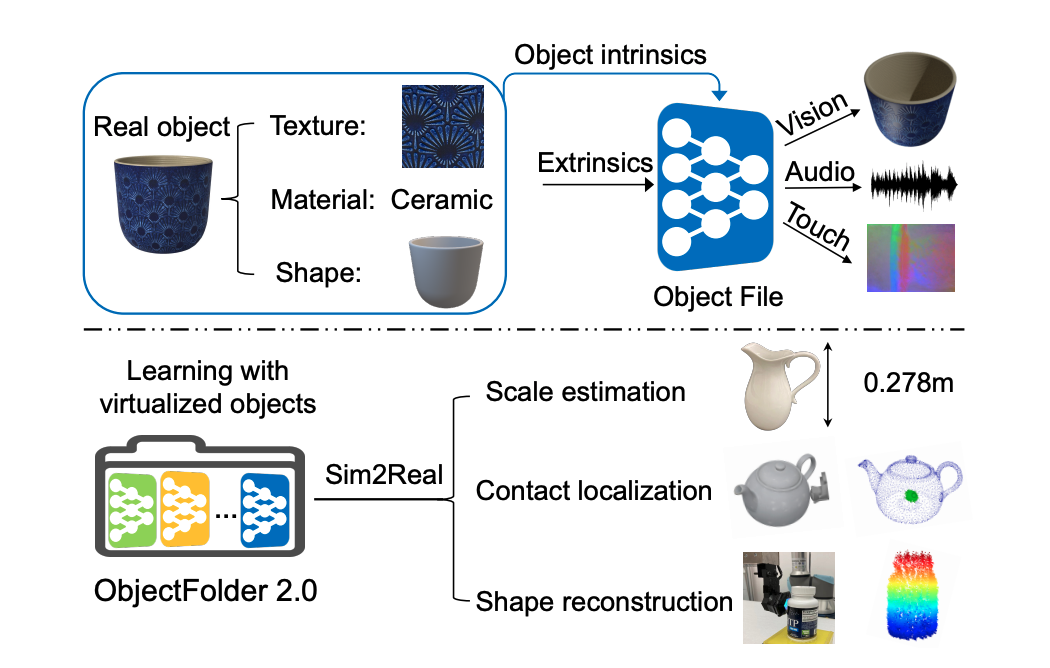
ObjectFolder 2.0: A Multisensory Object Dataset for Sim2Real Transfer
CVPR 2022
New Orleans, LA
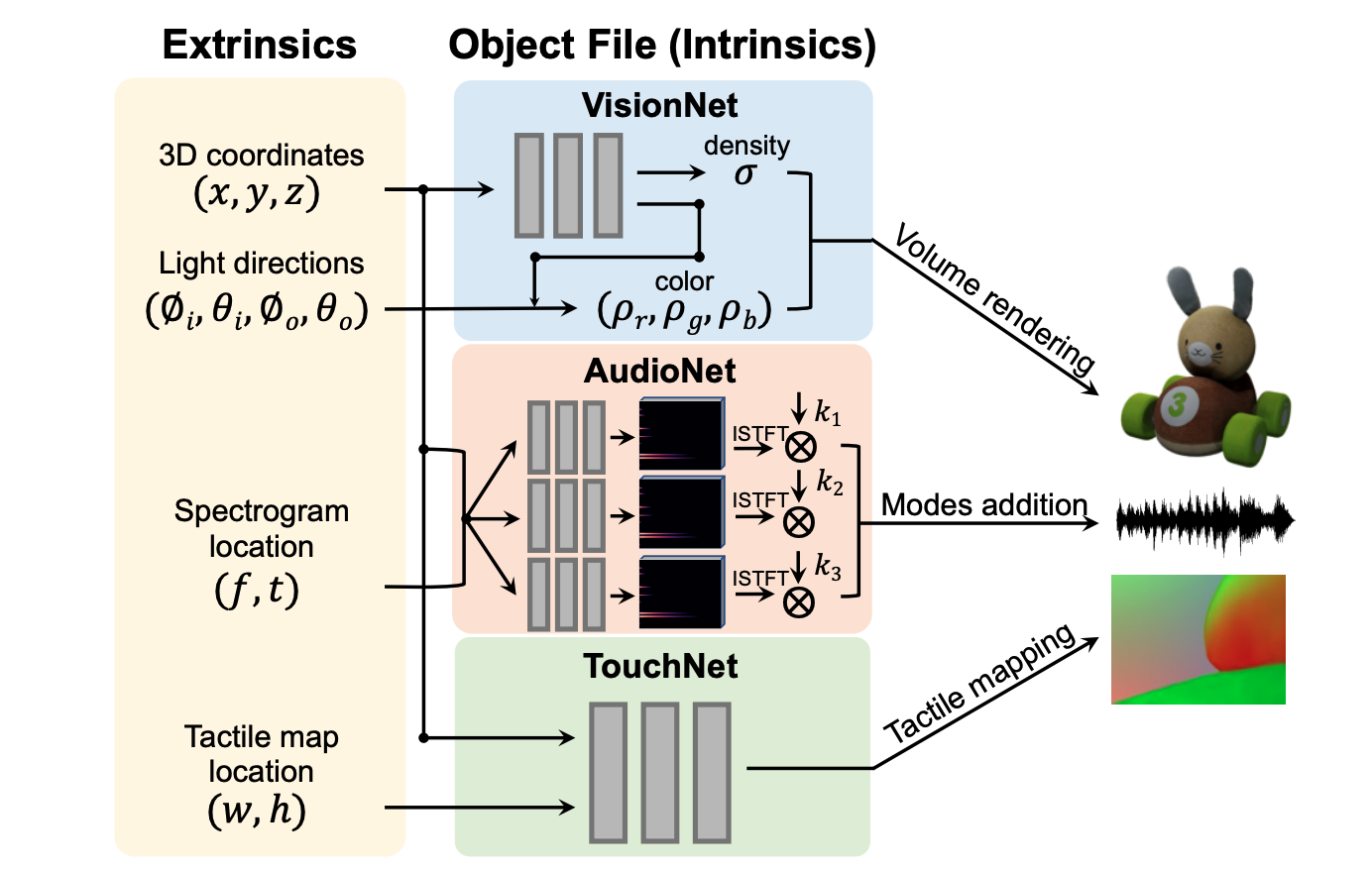
ObjectFolder: A Dataset of Objects with Implicit Visual, Auditory, and Tactile Representations
CoRL 2021
(Virtual)
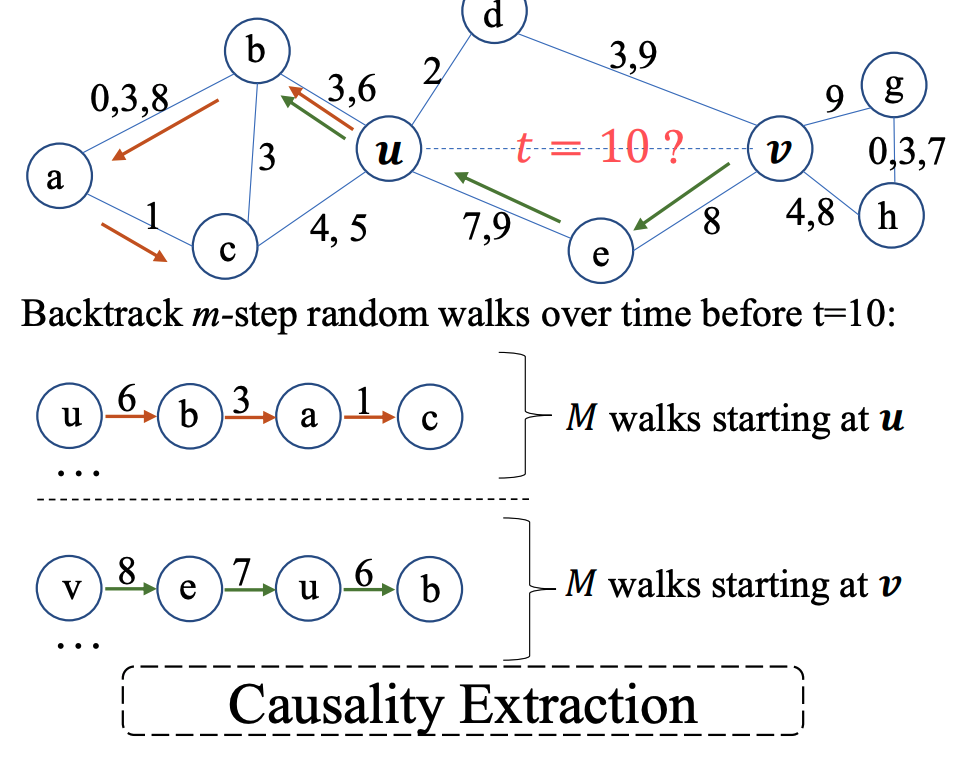
Inductive Representation Learning in Temporal Networks via Causal Anonymous Walks
ICLR 2021
(Virtual)
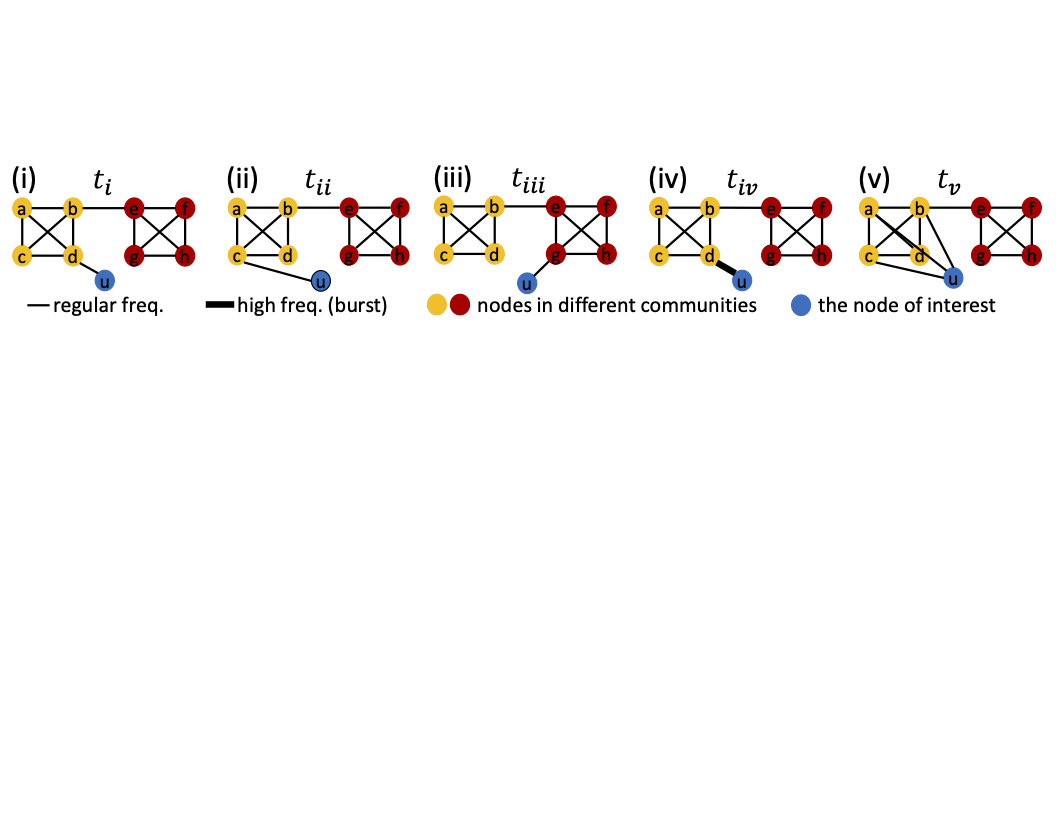
F-FADE: Frequency Factorization for Anomaly Detection in Edge Streams
WSDM 2021
(Virtual)
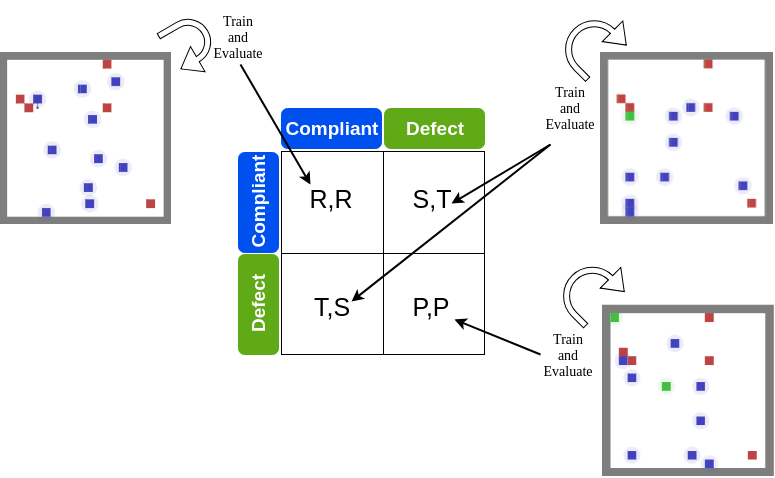
A Regulation Enforcement Solution for Multi-agent Reinforcement Learning
AAMAS 2019
Montreal, QC
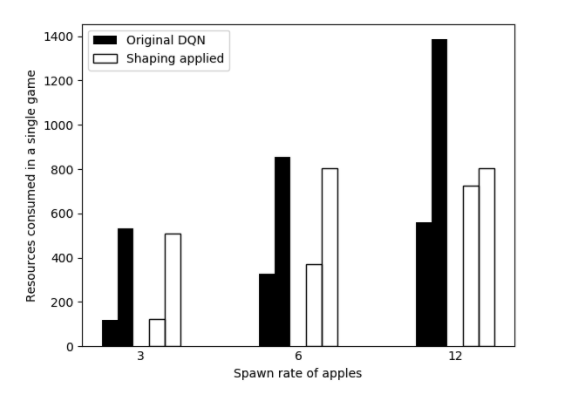
Designing Non-greedy Reinforcement Learning Agents with Diminishing Reward Shaping
AAAI/ACM conference on AI, Ethics, Society 2018
(Oral)
New Orleans, LA
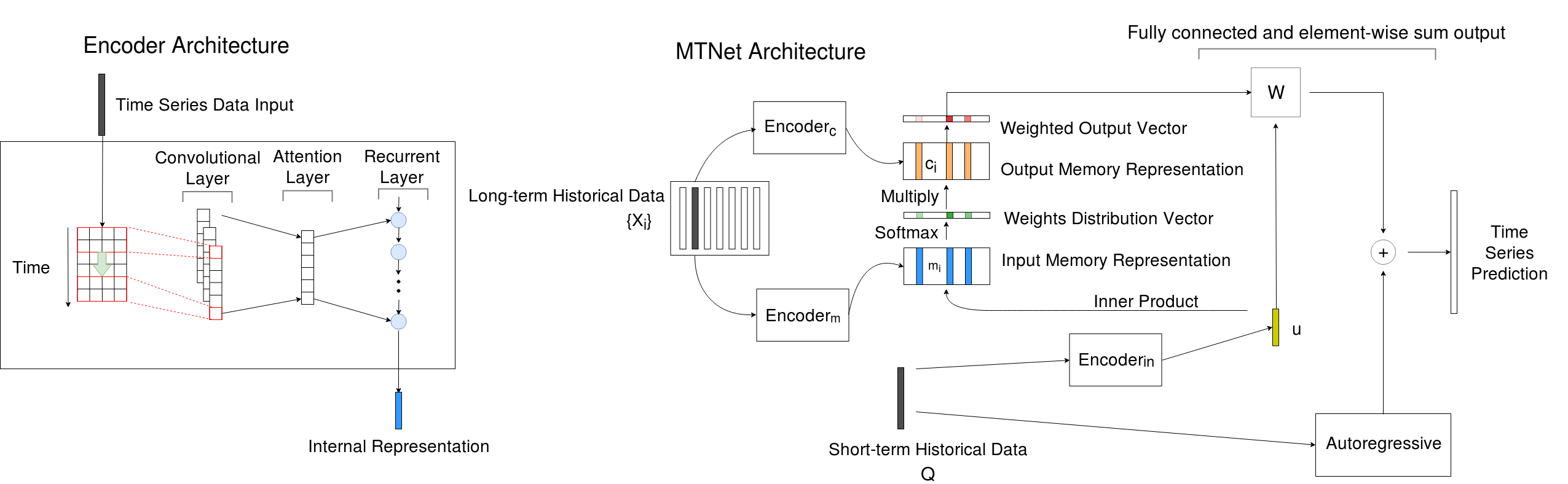
A Memory-Network Based Solution for Multivariate Time-Series Forecasting
preprint
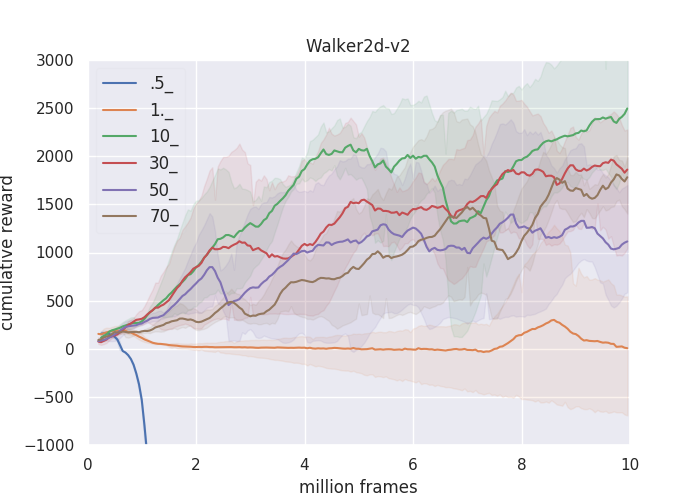
ANS: Adaptive Network Scaling for Deep Rectifier Reinforcement Learning Models
preprint
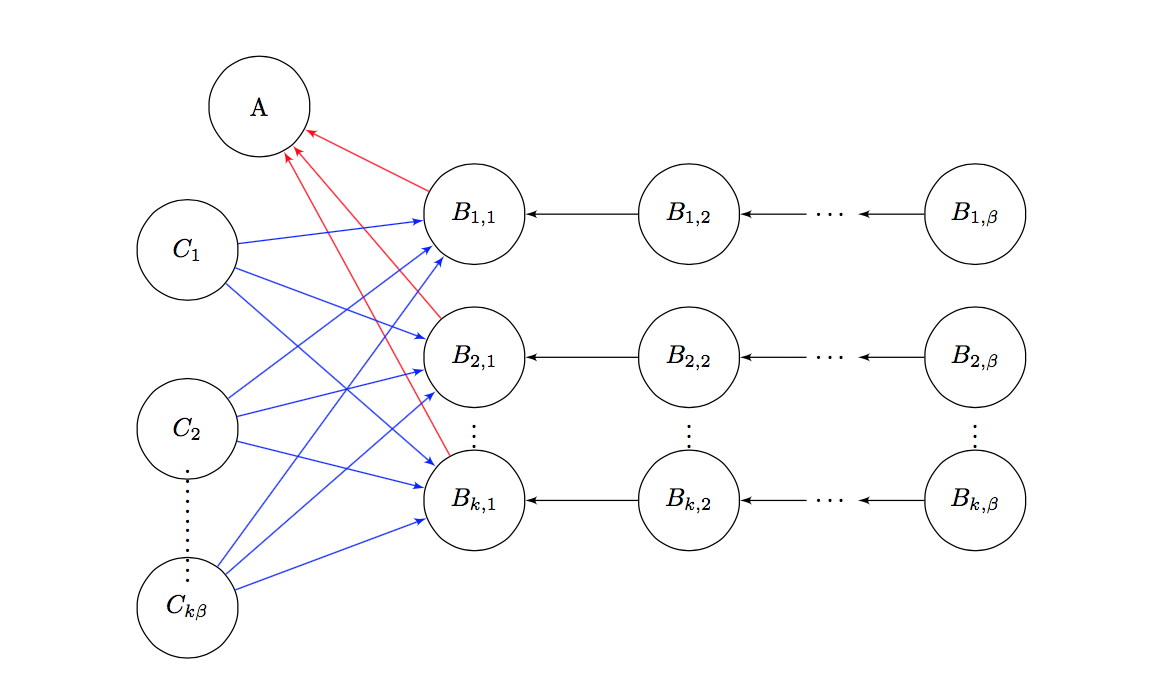
Heterogeneous Star Celebrity Games
preprint
Honors & Awards
- Ranked 19th (out of 4180) / KDD CUP - Main Track / 2018
- Ranked 4th (out of 4180) / KDD CUP - Specialized Prize for long term prediction / 2018
International
- Dean's List / National Taiwan University / 2016
- Finalist (Top 30) / International Physics Olympiad Domestic Final / 2013